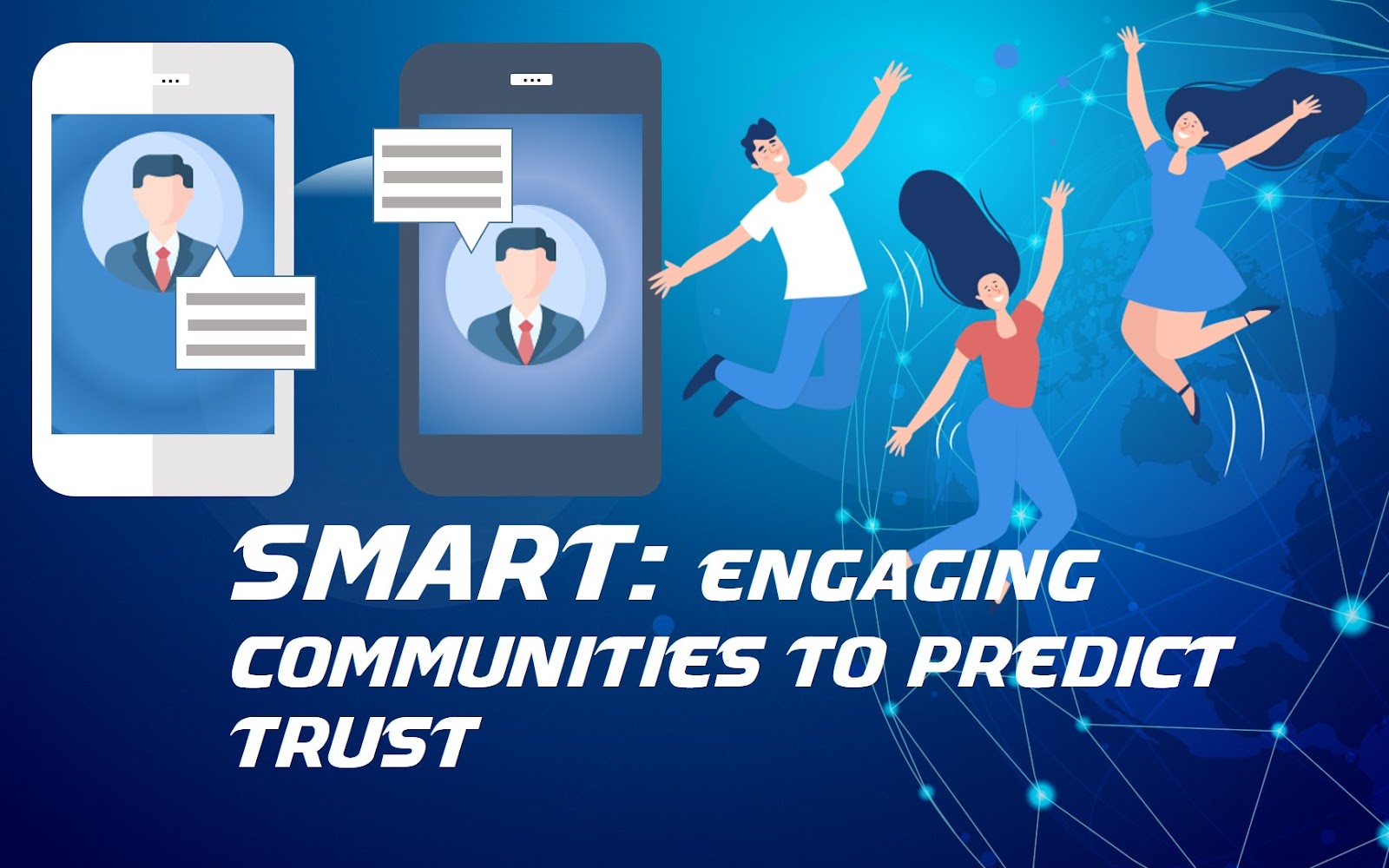
Trust challenges in social media
Social media platforms gained prominence as an essential technology for connecting people. Social media are centralised platforms that allow individuals to create, publish, and share content across an interconnected network. Additionally, social media are popular media for sharing information and news all over the world. Easy and quick information proliferation is one of the reasons for its popularity. However, such scenarios create an attentional bottleneck. It enables favouring information that is more likely to be searched for, attended to, comprehended, encoded, and later reproduced.
Moreover, the last decade saw an increasing engagement of people worldwide with online social media, regardless of age, gender, or nationality. Social media networks are a simple, fast, and attractive medium for sharing and transferring information. Moreover, people in different age groups, gender identities and societal beliefs engage in social media websites. This diversity poses critical issues regarding trust over the created content and the authenticity of those who publish it. It leads to fast data scattering at a high rate with minimal effort, enabling widespread malicious and untrustworthy content, harmful to society and individuals.
Such issues are vital when fake news, cyberbullying, and misinformation is regular occurrences across popular social platforms like Facebook and Twitter. Moreover, the integration of privacy-by-design features in social media platforms – such as anonymised identity systems that enable users to control their digital identity – further aggravates this problem. While such platforms improve regarding privacy violations, they pose traceability challenges, for example, in identifying who is publishing illegal, fake or malicious content.
Better social media platforms require innovative decision-making solutions at the level of individual engagement (i.e., content creation, propagation, consumption) and the underlying infrastructure. To prevent malicious information propagation in social networks, improving trust and reputation management systems is necessary, making them more inclusive. Integrating individuals who use social media in decision-making facilitates trustworthy, authenticated content creation and consumption. Additionally, it empowers people to tackle disinformation and fosters a positive engagement with fast-evolving digital technologies.
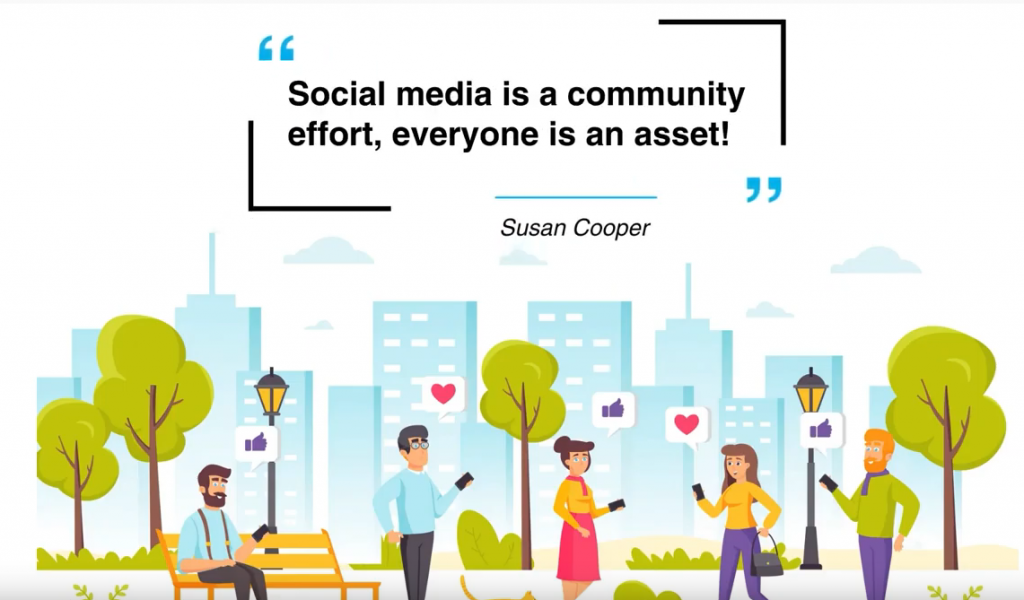
SMART: A ray of hope in the Dark Infodemic Age
ARTICONF develops a SMART tool that integrates technologies to improve trust and identify malicious actors in participatory exchanges across social media platforms. Essentially, it provides abstractions to characterise diverse sets of individuals using social media, maintaining traceability and ownership across the network without violating privacy principles. Essentially, SMART focuses on improving the quality of implicit and explicit trust-based communication across social media through collaborative decision-making for trust estimation and individual reputation computation.
Collaborative decision-making engages community experts and uses machine learning (ML) techniques to compute content trust metrics and classify them as real or fake.
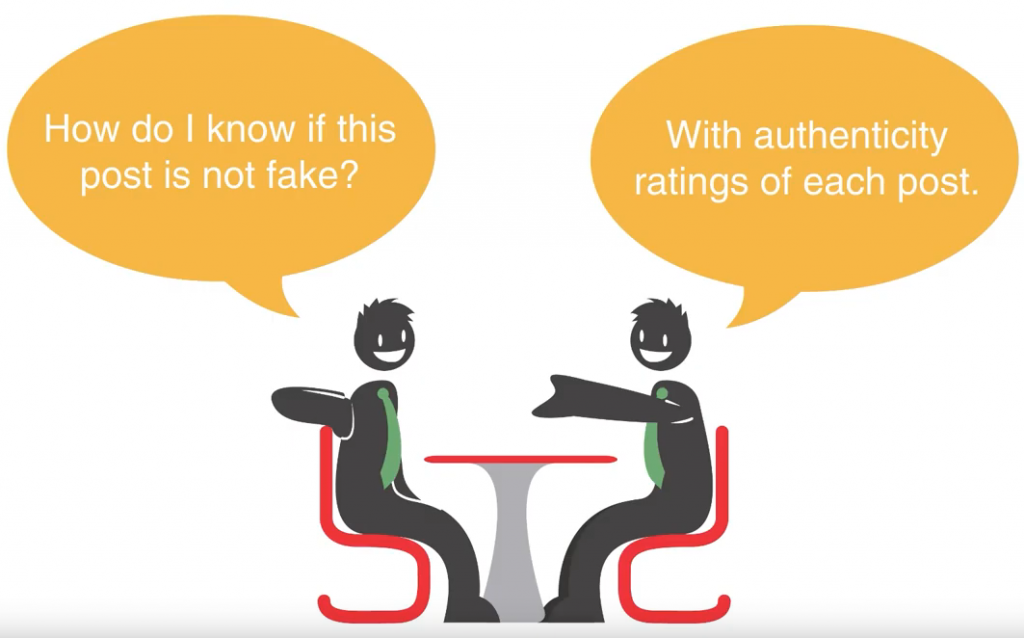
Individual reputation computation based on a rescaled sigmoid modelling of natural growth and decay rates in a non-deterministic environment (such as social media networks) prevents infinite trust accumulation by individuals.
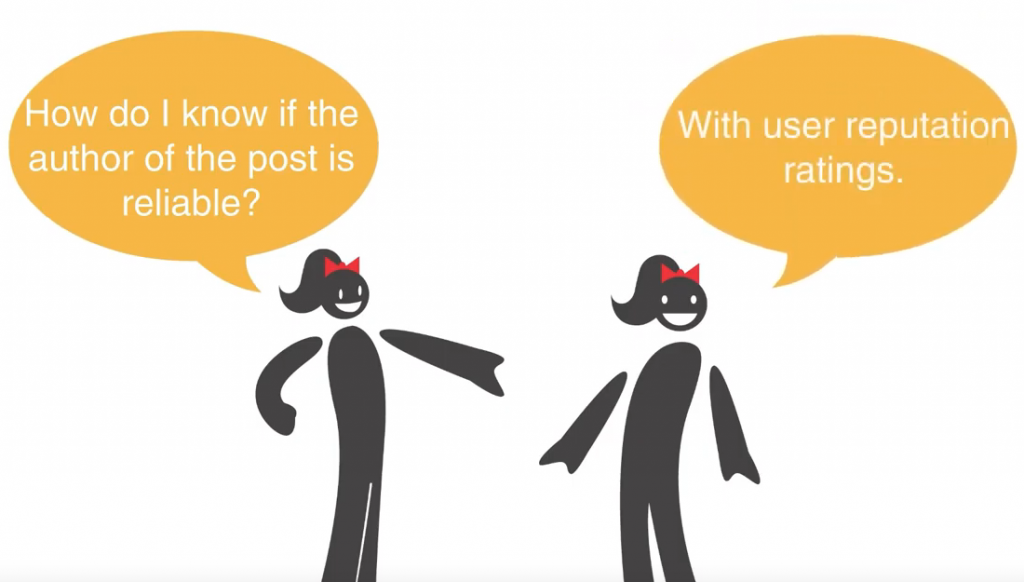
SMART’s data-driven approach and integrated design adopt a set of expert systems with a unique inference logic for estimating the trust of diverse social media content. SMART provides a list of trust oracles to all social media participants, representing expert systems with specific knowledge bases. The community members can choose one or more trust oracles by voting-based consensus to calculate intermediary authenticity metrics for each piece of content using a particular inference logic. What this means in practice is that each community member can vote on one or a combination of available trust oracles. The combination majority votes add up the results of the trust computation. Afterwards, SMART computes the weighted average of the trust ratings obtained from each oracle and labels content as trustworthy or fake. Finally, SMART aggregates the intermediary trust values of all content created by an individual and generates its reputation metric. Such a design allows SMART to provide fair and democratic decision-making for trustworthy content management.
SMART’s Trust Model
SMART initially computes the trust ratings using a set of oracles with their unique inference logic. Furthermore, it associates the average normalised trust ratings from different oracles to the content. A positive trust indicates trustworthy content, while a negative value suggests the opposite. SMART currently supports the two following types of trust oracles by design:
- Community voting based oracle utilises the percentage of upvotes gathered by content in a specific community to compute its trust rating. This oracle only considers voting performed by votes from the registered members of the community.
- ML-classification based oracle consists of binary machine learning models that classify content as trustworthy or fake. To achieve this, SMART developed a two-phase benchmarking model named WELFake. It is an ML classification model based on word embedding techniques and utilises linguistic features for fake content detection. The first phase validates the social media content using linguistic features. In contrast, the second phase merges those linguistic features with word embedding techniques and applies voting classification to generate an unbiased classification output.
SMART’s Reputation Model
SMART computes the reputation rating of an individual and classifies it according to trustworthiness or maliciousness in three stages.
Intermediary Reputation is the first stage that initially gathers the trust ratings of all content created by a user in a particular community. Essentially, each content created by the user varies in quality and authenticity and contributes to the intermediary reputation differently. Hence, SMART utilises content volume (measured in the number of characters) to distinguish the quality of different content. SMART assumes that extensive and explicit content contributes more significantly to the user reputation.
Local Reputation is the second stage that represents the trustworthiness of a user in a uniquely identified community. In this stage, SMART initially combines the intermediary reputation ratings and computes the local reputation of users in a community using a rescaled sigmoid technique. Additionally, it utilises a reputation threshold decided by community members via consensus to classify a user as trustful or distrustful.
Global Reputation is the third and final stage and reflects the accumulated trust ratings of a user across all the communities of a social application. In this stage, SMART gathers and averages the local reputation of a user across all communities.
What’s in store for the Future?
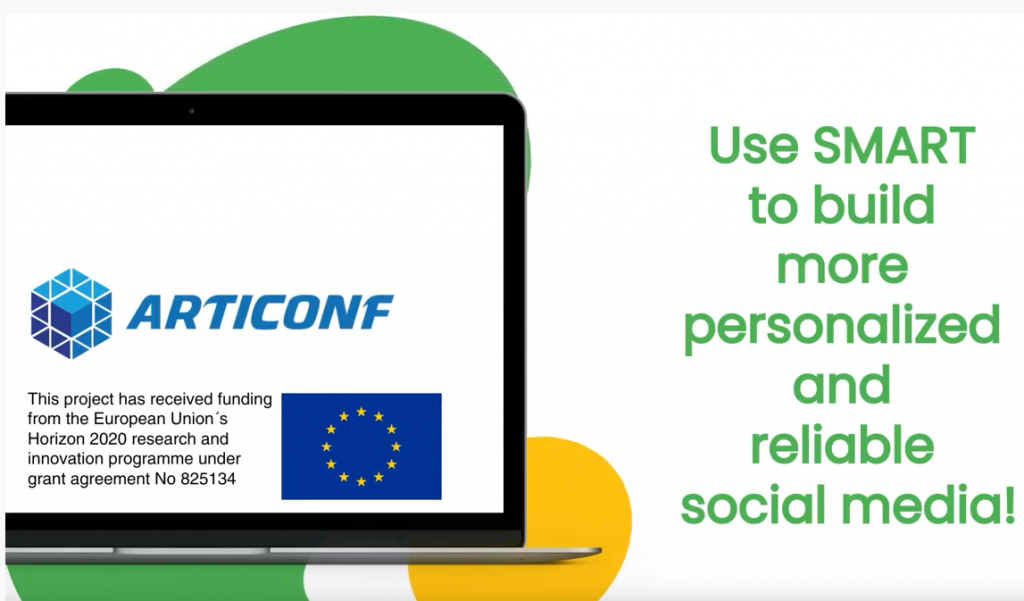
By December 2022, we plan to integrate online fact-checkers to the SMART tool to improve fairness across computation of authenticity and reputation ratings. We also aim to validate the trust and reputation management for ARTICONF’s use cases and other business-oriented social media applications. To learn more about SMART and integrate it into your decentralised social app environment, please check the SMART open-source GitLab repository.
This blog post was written by Alpen-Adria-Universität Klagenfurt team in October 2021.
< Thanks for reading. We are curious to hear from you. Get in touch with us and let us know what you think. >