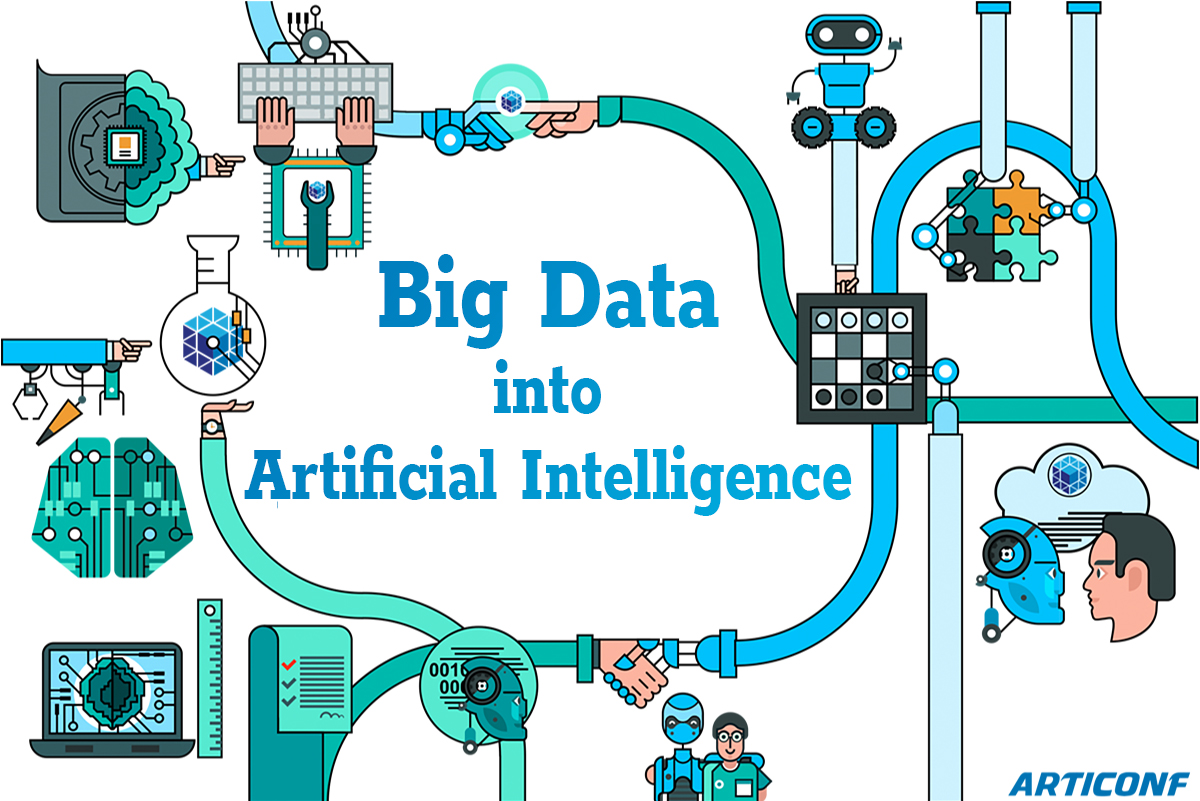
An ARTICONF Future Vision
Big Data promises to transform Artificial Intelligence (AI) by providing immense volumes of data. The vision of Next-Gen Big Data Intelligence represents the main ingredient that fuels AI platforms and contributes to unleash its huge and still unexploited potential towards mass production and pinpoint consumption, with tremendous influence on our society.
Such impacting technology needs to provide transparency, fairness, trust and reduce bias in providing Big Data Intelligence. Unfortunately, the current Big Data technologies diverge from this path, where AI platforms typically operate within centralised proprietary organisations that manage, and control them, exposing critical issues of AI algorithmic [1] and data [2] biases, accompanied with insidious and pervasive reinforcement of discriminatory societal practices. This is particularly problematic when training AI models using raw, noisy or inadvertently biased data tainted by deep-rooted societal discriminations [3] (e.g. Amazon Rekognition, Google hate speech detector) is a regular phenomenon at the hands of centralised intermediaries.
To better understand Big Data and AI problems, we illustrate some of its challenges through an ARTICONF’s (EU H2020 project on Decentralised Social Media Platform) car-sharing use case, provided by an SME Agilia Center SL. Currently a partner in ARTICONF, AGILIA provides mobility-as-a-service (MaaS): where car owners rent their vehicles while travellers can book their trips with advantageous offers. Additionally, AGILIA relies on anonymized traveller data gathered through its own social platform to understand customer preferences and provide a better experience through AI-driven technologies. For such purposes, AGILIA requires a large amount of data not only from its own social platform, but also from diverse MaaS providers (e.g. Uber) for analysing a wide spectrum of data. With such goals, AGILIA envisions transforming their use case with AI-as-a-Service support to provide unbiased intelligent analytics for a range of stakeholders (e.g. MaaS providers, Car insurance companies etc.). However, such vision comes with its own set of challenges. The following section enumerates a few of them, trying to understand how this affects AGILIA’s visions.
- The first and foremost issue lies in the presence of centralised and unaccountable AI solutions. Typically, solutions towards AGLIA’s goal of providing value-added analytics rely on AI platforms owned by single proprietary organisations (e.g. Google, Amazon, Nvidia) that control user activities and access to the AI services on the market. This leads to a lack of transparency and interoperability, hindering the participation of smaller organisations in data-driven innovation. The centralised nature of the AI platforms and algorithms owned and operated by big companies that collect and control large-scale volumes of data raise privacy, ownership, scalability and accountability problems. Users have little control over the “intelligent” solutions, which are easily subject to manipulations. The algorithms utilised by these platforms are therefore questionable and lack means to interpret, explain and trust the solutions.
AGILIA Scenario: Several car insurance companies would utilise the AGILIA vehicle mobility platform to predict prices involving multivariate parameters across various routes (e.g. driving risks, dangerous areas, demand). In such scenarios, there are risks in collecting demographically biased data. For example, ride-hailing drivers may intentionally choose not to drive in certain areas, falsely recognised by the AI algorithms as dangerous routes, leading to discriminatory mobility prices for given demographic regions and violating the cost-efficient principles of the MaaS paradigm. - Another issue lies in the costly integration of data. AGILIA relies on the availability of valuable data. Currently, most data platforms are proprietary silos that do not consider the possibility of data sharing at the creation stage, owing to security and data rights management concerns. Furthermore, large-scale data originating from disparate and untrusted sources raise complex variety and veracity challenges, leading to costly integration and validation. This inhibits multilateral interoperability across vertical, cross-sectoral, personal and industrial data spaces, hindering the sustainability of data sharing ecosystems.
AGILIA Scenario: AGILIA’s vehicle mobility platform vision promises to utilise shared services and provide benefits to consumers through optimised journey planning and pricing packages. However, the mobility data required to improve customer convenience lies in the federation of segregated Service Providers (e.g. traffic conditions, weather information, on-board video streaming and data silos). The absence of a unified platform to share this data, currently administered by fragmented providers, causes AGILIA to become wary of security and ownership compromises. This raises governance issues related to privacy, integration and validation of sensitive personal data (e.g. associated with the routes of vehicles or to the practices of passengers and drivers).
While solving AGILIA’s aforementioned challenges for its visionary MaaS platform is beyond the scope of the ARTICONF project, which primarily focussed on creating a decentralised platform for EU Research and Innovation Action ‘Future Hyper-connected Sociality’ [4], and doesn’t necessarily deal with Big Data and AI problems. However, ARTICONF does lay down the ground rules for creating a transparent Big Data and AI platform through the following principles.
- Democratization of AI: ARTICONF provides opportunities for its stakeholders to be a part of a data-driven decision-making process across the decentralised network through the application of federated and transfer learning mechanisms. While such AI technologies are partially democratic and can help AGILIA eradicate some of the algorithmic AI biases, they are still far from completely democratizing AI. However, steps in this direction can be taken through the decentralisation of AI networks using emerging decentralised ledger technologies (DLTs). First, such platforms can stimulate diverse stakeholders to share performant and interoperable AI models. Second, it can allow assessing AI model’s trustworthiness to detect algorithmic and data biases by tracing time stamped and signed immutable AI transactions, and linking AI models to the training, testing and inference datasets. However, current DLTs are still unable to handle computationally costly AI processing.
- Data integration through federation: ARTICONF’s decentralised architecture provides an adaptable Blockchain-as-a-Service platform to federate DApps from diverse domains. This also provides an opportunity to federate data from multiple data providers (e.g. MaaS providers). Additionally, this improves data providers’ control with a secure, permanent and unbreakable link to their data, maintaining ownership and control. Moreover, it provides sustainable integration and validation across large-scale, high variety and veracity data, and is able to record and audit traceable information, including data origins (i.e. raw metadata). Such ARTICONF features could be utilised to provide provenance tracking of data for enhanced bias detection and present fairer and accountable AI-driven analytics.
There is no denial that Big Data and AI are some of the next generation technologies for digital services, however there is a need for those technological advancements to mature before we try to reap its benefits. ARTICONF will not solve all aforementioned challenges of Big Data and AI, though it envisions bringing such advancements to the fore.
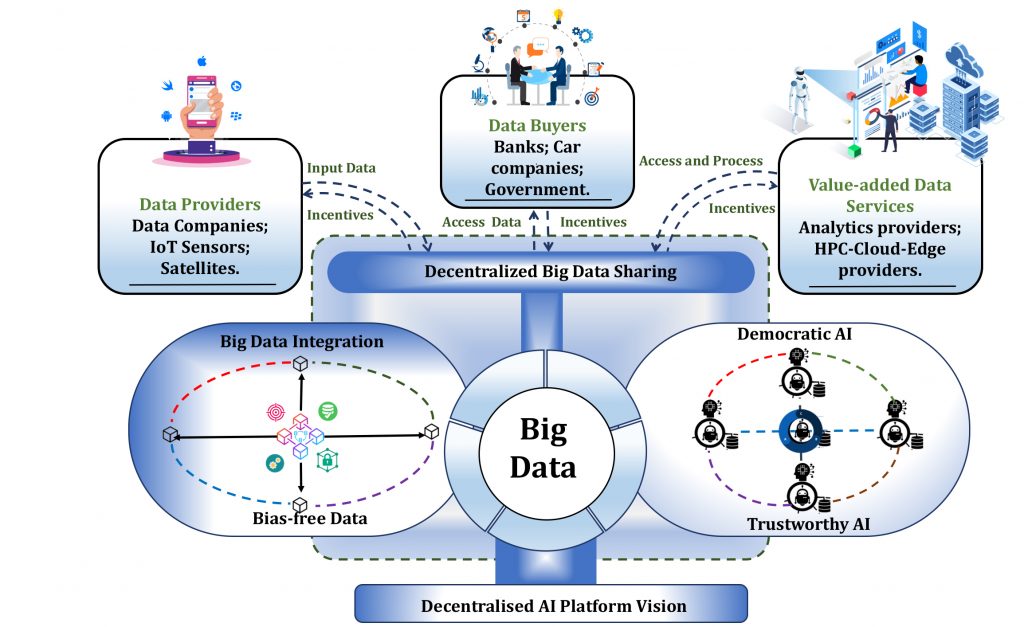
To conclude, such future vision could only be realised by understanding and answering the following prominent issues which are not only technological, but also governance related.
- Are our technologies mature enough to lower down the existing friction between Decentralised knowledge – centralised AI, where knowledge acquisition is intrinsically a decentralised activity, whereas the source of authority control in AI platforms is centralised?
- How can we optimize Transparency – stakeholder influence ratio, where centralised AI systems disproportionately influence the stakeholders’ decision-making ?
[1] AI Algorithmic Bias, https://www.technologyreview.com/s/612876/this-is-how-ai-bias-really-happensand-why-its-so-hard-to-fix/
[2] AI Data Bias, https://towardsdatascience.com/racist-data-human-bias-is-infecting-ai-development-8110c1ec50c
[3] AI Discriminations, https://www.lexalytics.com/lexablog/bias-in-ai-machine-learning
[4] EU RIA Action ‘Future Hyper-connected Sociality’, https://cordis.europa.eu/programme/id/H2020_ICT-28-2018
This blog post was written by Alpen-Adria-Universität Klagenfurt team in August 2020.
< Thanks for reading. We are curious to hear from you. Get in touch with us and let us know what you think. >